AI with Real-Time Data: Emerging Trends and Use Cases
AI with Real-Time Data: Emerging Trends and Use Cases
For many users, especially in developed regions, the days of waiting for websites or data transfers are becoming less frequent thanks to faster infrastructure and edge delivery. However, real-time responsiveness is still critical to ensure seamless user experience and operational performance. Consumers don’t only expect real-time data delivery; many technologies rely on it to function properly. Autonomous vehicles, smart cities, and financial institutions, for example, need real-time data to protect drivers, citizens, and customers.
Emerging trends demonstrate the power of this technology and can be a sign of more to come. This article covers the basics on real-time data, how AI assists its delivery, and where your business might expect to use this technology in the near future.
What Is Real-Time Data?
Real-time data describes the delivery of data, either immediately or with minimal delay, right after collection or creation. Its arrival should feel instantaneous to end-users, which allows them to start analyzing and taking action right away. This is opposed to data processing, where data is often collected and processed in chunks. With real-time data, users have access to a continuous stream of information.
Why Real-Time Data Matters in AI
Paired with artificial intelligence (AI), real-time data can make the technology more effective for users, improving rates of accuracy and enabling timely decisions with up-to-the-minute information. Instead of just reacting to information, real-time data and AI can be used together to improve predictive analytics capabilities and enhance user experience. When machine learning models are trained on real-time data, they can be significantly more accurate, morphing to fit new findings and identifying new patterns as they develop. For some businesses, immediacy is critical – delayed applications can impact revenue, key business processes, and even safety.
Five Ways AI with Real-Time Data Can Help in Business Settings
Organizations that can benefit the most from AI with real-time data are those that are looking for more innovative solutions and greater efficiency as part of overall digital transformation efforts. Here are five key ways this combination can help businesses:
- Smarter, Faster Decision-Making
Businesses can rapidly respond to changing market conditions, operational challenges, and customer demands with real-time AI. Processing and managing data efficiently allows for faster, more well-informed decisions. - Increased Productivity for Employees
Repetitive tasks can slow down employee productivity and make work more prone to errors. AI can automate these tasks, freeing up staff for more strategic work while empowering them with more thorough and accurate insights. - Greater Organizational Efficiency
Resource allocation, key processes, and operational efficiency can all be optimized with real-time AI with predictive maintenance, automated supply chain management, and analytics. - Enhanced Personalization
When customer data is processed in real time, personalized recommendations can also be made immediately. This can be done on the application or website, as well as in marketing messages. Making tailored recommendations can improve the customer experience and build brand loyalty. - Better Customer Engagement
Shorten the time to answer customer queries with real-time AI, providing feedback instantly through AI-powered chatbots that can deliver responses using sentiment analysis and other automated tools.
Key Technologies Powering Real-Time AI
Some key technologies work in concert with real-time AI to make it possible to process and react to data as it comes in.
The Role of IoT in Data Generation
The Internet of Things (IoT) includes sensors and devices that can be found on factory floors, in autonomous vehicles, and in smart homes, among other places. These generate massive data and collect real-world information, including location, temperature, moisture, and movement. The raw data collected by these devices informs real-time decisions.
Edge Computing for Instant Processing
With edge computing, data is processed closer to the source—such as sensors, cameras, or devices—at the network’s edge. This reduces latency and allows for faster decision-making, which is critical for time-sensitive use cases like autonomous vehicles or industrial automation.
Cloud Computing for Scalability
While cloud platforms offer essential scalability and integration benefits, some real-time AI workloads may still require edge or hybrid cloud deployment to meet latency and responsiveness requirements.
Data Streaming for Continuous Insights
Data streaming platforms process data in real time instead of in batches, creating a continuous flow of data that moves from the source to the point of analysis. With data flowing constantly, AI systems can find anomalies and identify trends more quickly, making predictions that allow for practically immediate action.
Real-World Applications of AI with Real-Time Data
Real-world applications for AI and real-time data can be found in many different industries, but here are a few common use cases where this technology can improve user experience, patient outcomes, consumer safety, and more.
- AI-Powered Healthcare Systems and Diagnostics: Real-time analysis of patient data in intake forms and imaging has the potential to transform the patient experience when used responsibly. Real-time data analysis from remote monitoring devices can help medical practitioners note critical changes in patient vital signs outside of the office, giving them the chance to intervene quickly. AI-driven image recognition in medical imaging can potentially speed up diagnosis and improve accuracy. However, these systems must be validated rigorously, meet regulatory standards, and mitigate bias from limited or non-representative training data before being deployed in clinical practice. Real-time analysis of patient data can help create tailored treatment plans and build predictive models for possible disease progression. Finally, real-time data enables remote patient care through telemedicine systems.
- Financial Services and Algorithmic Trading: Financial scenarios can change in an instant. ML models can use real-time market trends to identify and recommend changes to mitigate financial risks. Rapid market trends can also be acted on by AI algorithms that can execute trades in an instant. Real-time data delivery can help financial organizations identify suspicious patterns in activity, detecting fraud faster to improve customer safety. AI-powered chatbots can also use real-time data to deliver customized financial advice.
- E-Commerce, Retail, and Consumer Insights: AI algorithms can create a truly personalized customer experience, providing individualized recommendations for what to purchase next, providing instant support via chatbots based on customer queries, and tracking inventory and sales data instantaneously. These technologies can also adjust prices based on demand, competitor pricing, and more.
- Autonomous Vehicles and Transportation: Sensor data from LiDAR, radar, and cameras in autonomous vehicles are only effective with real-time responses. This way, cars can navigate complex environments and avoid obstacles with ease. Autonomous vehicles and other transportation methods can also use real-time monitoring to anticipate potential failures in vehicle systems that can aid in predictive maintenance instead of relying on reactive fixes. Real-time data can be used in ride-sharing applications to dispatch vehicles effectively, and real-time analysis of traffic patterns can help automobiles make dynamic adjustments to their routing.
- Smart Cities and Intelligent Infrastructure: Understanding how energy is consumed in real time can help smart cities optimize grid performance and reduce waste. Smart cities can also use environmental monitoring, real-time analysis of air and water quality data, to quickly respond to pollution events. Safety can also be improved through the use of real-time video analysis and sensor data, improving the ability to complete surveillance tasks. Some municipalities are beginning to pilot real-time infrastructure monitoring with embedded sensors and AI analytics, aiming to detect early signs of wear or failure in roads and bridges.
Considerations for Incorporating AI with Real-Time Data
When a business starts incorporating AI and real-time data into its processes, data privacy, scalability, integration, and employee training need to be top-of-mind.
- Prioritize data privacy and security measures: Don’t compromise security in the name of rapid delivery. Businesses should implement robust access controls, encryption measures, and data anonymization to protect the privacy of users and devices submitting sensitive information. Regularly audit security measures to find and address vulnerabilities. These audits should also include evaluations of current compliance with relevant data privacy regulations specific to the organization.
- Assess scalability, processing, and infrastructure limitations: Collecting, processing, and storing real-time data with AI demands a lot of resources. Businesses using these technologies should consider how their workloads will scale in the coming years and have a plan in place for infrastructural upgrades that also satisfies bandwidth and latency requirements.
- Have a documented plan for integration with current workplace tools: Making data delivery and analysis more efficient will only work if your existing workflows and systems can be integrated with AI. By understanding the potential impact on current applications and databases, you can implement integrations or make plans to upgrade legacy systems over time.
- Communicate best practices and expectations for use to employees: For employees who are unfamiliar with AI and real-time data, they may have misaligned expectations for what the technology should be able to do. Provide comprehensive training on how AI tools work and how employees can interpret the generated insights. Businesses should also establish clear guidelines for how data should be used and how to engage in responsible AI practices.
Best Practices for Maximizing AI with Real-Time Data
To get the most out of AI and real-time data, including the greatest accuracy, efficiency, and security, organizations must keep these best practices in mind.
- Build a robust data pipeline: A reliable and efficient data pipeline can make integration, delivery, and processing more efficient. Implement data streaming technologies that can handle continuous data flows while keeping latency low. Data cleansing, validation, and transformation processes will keep data quality high. Finally, automate where you can to improve accuracy and scalability while minimizing the frequency of manual intervention.
- Strengthen data governance and compliance: Defining data ownership, access controls, and usage guidelines as part of your data governance policies can protect your data and workflows while allowing certain groups and individuals the access they need to form critical insights. All real-time data and AI processes need to abide by relevant data privacy regulations for your industry or particular business, such as CCPA or HIPAA. Regularly review and update these policies to keep up with changing regulations and business needs.
- Invest in scalable AI infrastructure: Cloud-based infrastructure can improve the flexibility and scalability necessary to operate real-time AI applications. Edge computing can lighten the burden on a central data center by processing data closer to the source, improving responsiveness and decreasing latency. Businesses may also want to consider distributed computing architectures to handle the large volume of data created by real-time AI applications.
Use AI with Real-Time Data to Accelerate What’s Next
Is your data strategy keeping pace with your business goals? Many organizations struggle to unlock the full value of their data—especially when it’s not collected, processed, or acted on in real time. Whether you’re modernizing legacy systems, optimizing infrastructure for AI, or scaling to support real-time insights, TierPoint can help.
Our Advisory and Consulting Services bring the technical expertise and strategic guidance needed to build a resilient, scalable data ecosystem—across cloud, edge, and hybrid environments. See how innovators like Groq are using real-time AI to transform performance, and explore practical steps for your organization in our latest white paper on accelerating business outcomes with AI.
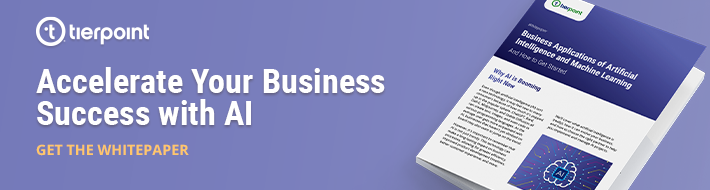
More >> AI with Real-Time Data: Emerging Trends and Use Cases